The prevalence of diabetes is increasing worldwide, and its associated long-term complications involving tissue damage and organ failure places an additional on the healthcare system [1]. In the UK, the number of people living with diabetes doubles in twenty years, according to the recent report by the British Diabetic Association.
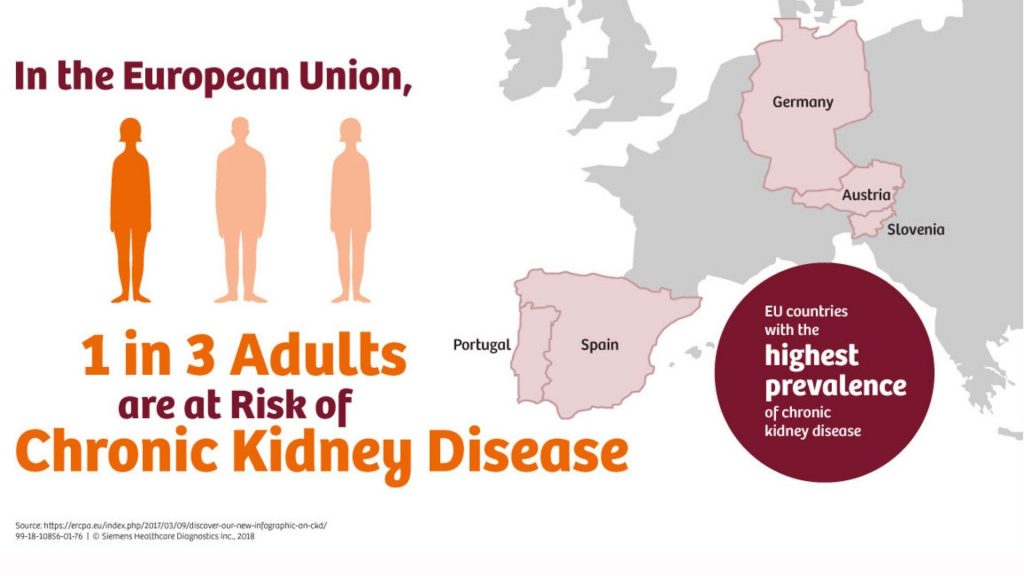
Diabetes therapy is complex. Timely diagnosis, self-management and continuous medical care are required to prevent both chronic (e.g. retinopathy, nephropathy) and acute complications (e.g. ketoacidosis). Therefore, therapeutic decisions need to take into account diverse lifestyles and medical-related factors that must be optimised to enhance the quality of life. Amongst microvascular complications, diabetic neuropathy is the leading cause of end-stage renal disease and has a high cardiovascular risk. People with diabetes are five times more likely to need either kidney dialysis or a kidney transplant. Identification of these risk factors as well as a timely diagnosis is of paramount importance for effective treatment
In the past algorithms have been developed to predict the diabetes complications. Huang et al. developed a gender-based rule in a decision tree-based model integrating genetic and clinical features to distinguish diabetic nephropathy with non-DN among 345 Taiwanese T2D. Using a five-fold cross-validation approach with a decision tree, random forest, libsvm, and naïve Bayes approach, found to generate the best performance. Makino et al. used AI constructed prediction for diabetic kidney disease (DKD) using time series and logistic regression. The new model able to detect the progression of DKD with 74% accuracy. Dagliati et al. used logistic regression along with NB, SVM, RF with stepwise feature selection to predict the onset of retinopathy, neuropathy or nephropathy at 3, 5 and 7 years among 1000 T2DM patients. Data were imputed by using statistical method and RF and compared its performance using RMSE, RMSEN while validation carried with a leave-one-out (LOO). Sensitivity, specificity, PPV, NPV and AUC and MCC were measured and approach found 86% accuracy. Still, the accuracy and validity of these algorithms need further improvisation. Both diabetes and DM are caused by multiple risk factors that include the interaction between specific genes and environmental factors.
In the UK around 40% of people with diabetes develop nephropathy and the total annual costs to the National Health Service (NHS) of managing DN were US $231 million US$231 million (£152 million) for Type 1 diabetes (range: US$190–350 million [£125–230 million]), US$933 million (£614 million) for Type 2 diabetes (range: US$809 million–US$1.4 billion [£532–927 million]), and US$1.2 billion (£765 million) for all diabetes (range: US$999 Million–US$1.8 billion [£657 million–£1.2 billion]).
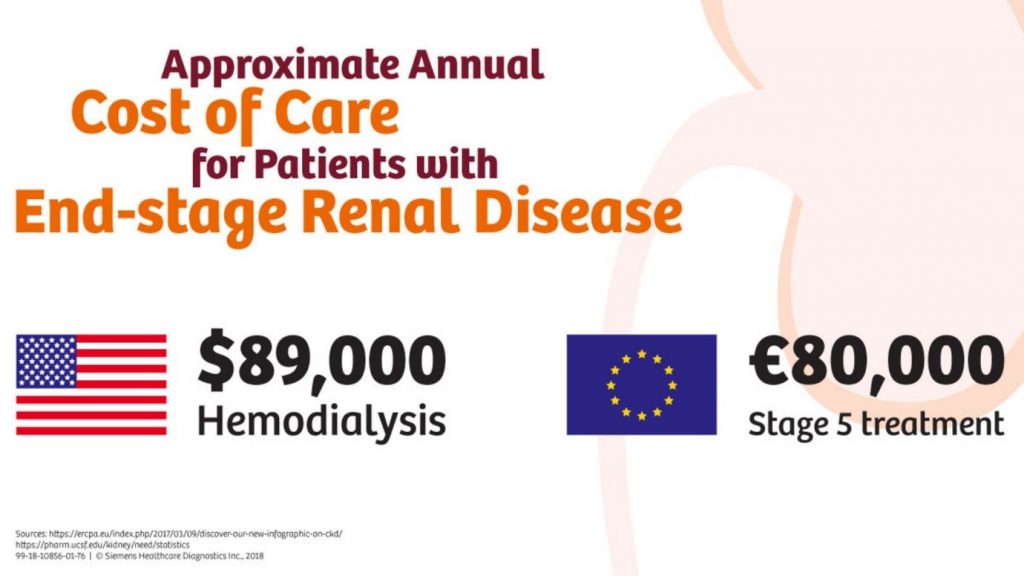
But it is possible to prevent or delay diabetes related complications if high-risk groups are identified at the right time and treated with the right approach. A machine learning algorithm does wonders where it helps to identify high risk population.
Contact us for more information about how we can help you to use your EHR data or sensor data to identify high risks patients using ML.
Comment here